Everybody Lies, What the Internet Can tell us about who we really are, by Seth Stephens-Davidowitz
Seth Stephens-Davidowitz is an economist and data scientist. He uses data, particularly massive data about google searches, to draw conclusions about human behaviour.
I loved this book, and to the frustration of my family, I kept reading bits out to them as I worked my way through it.
Probably the core of it is the way in which google searches are, themselves, information.
One of Stephens-Davidowitz’s early papers (2013), for example, looks at the use of racially charged language in google searches (the n-word) as a proxy for whether people are racist. He then demonstrates that a higher than average number of this type of search is a predictor of lower than expected voting for Obama in the 2008 and 2012 elections (after controlling for John Kerry vote in 2004). That is the one of the most charged example of the way in which google searches reveal people’s real thoughts (rather than what they are willing to reveal in surveys, or polls).
As a book, it is a selection of fascinating research about the human condition; some confirming our biases with new, different information, and some quite counter-intuitive using a variety of massive data sets. For example:
- People in warm climates tend to be less depressed than people in cold climates
- Parents are more likely to think their son is gifted than that their daughter is gifted
- Australian women are most worried about eating cream cheese during pregnancy, whereas Singaporeans are worried about whether they can drink green tea, and Nigerians are worried about drinking cold water
- Prisoners who are assigned to harsher conditions in prison are more likely to commit crimes on release
- Baseball team preferences (for US men) are strongly influenced by the team that won the world series the year they turned 8
- Superbowl advertising actually is worth while despite the immense cost
For me, just reading these snippets, and the immensely clever and convincing ways Stephens-Davidowitz goes about demonstrating them with a wide variety of data sets made the book worth while.
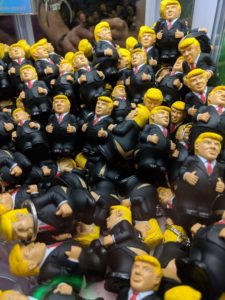
Is there more to it, though? Probably the more worthwhile part of this book is the warning section – Big Data – handle with care. Stephens-Davidowitz first of all points out what should be obvious to anyone interested in financial markets – there is rarely money to be made in the stock market from this kind of analysis. A trading insight is quickly copied, and the information added to market prices. And then there are some complex systems where the interactions and potential variables are just to large to be teased out even by the biggest dataset. For example, DNA. For a while, there was a trend for geneticists to discover traits that were coming from different individual parts of DNA. But the human genome is unbelievably complex, with interactions with the environment that mean that teasing out the function of an individual aspect is still mostly beyond our reach.
Big data (just as most forms of data analysis) also creates an over emphasis on what is measurable. Proxy variables that can be measured stand in for what would ideally be measured but where data doesn’t exist. For me one of the pernicious examples of this is in educational testing. Governments everywhere would like to measure the effectiveness of education, and improve it. So many governments (Australia is one with Naplan) create educational tests as a proxy for whether children are being appropriately educated. And then perverse incentives are created which mean that Australian children are educated very well in how to do well on the Naplan tests.
That doesn’t invalidate the Naplan as a concept, but it is always important to remember that the metric is just what is being measured; not necessarily the effect that you want to be measured.
And then finally, just because you can use big data, doesn’t mean you should. Big data generally gives you probabilities not certainties. The famous article about Target predicting a teenage girl’s pregnancy and sending her ads for baby products and outing to her father is actually a myth (this article shows how it grew out of a number of people telling the story in turn and making it bigger and better). But should a company use their data in this way as a predictor of behaviour? What about a government? It probably depends on the consequences of getting it wrong. Sending someone an ad for baby related products is not that disastrous. But deciding on whether someone deserves parole, based on their probability of reoffending? That’s something to take a lot of care with.
So should you read this book? If you like stories of analysis based on clever uses of data, absolutely read it.
It’s not a how to book, but it does give you a sense of what is possible, and Stephens-Davidowitz’s website gives you lots of resources if you’d like to learn more. It certainly made me want to play with some of the data sets available, even though there is quite a lot I would need to learn about large-scale data manipulation to perform the kind of analysis that seems effortless in this book.
I saw a definition the other day: Big Data – statistics done by non statisticians. Probably sums up the tendency for inferences and trends to become “facts” in the wrong hands!