Escape from Model Land: How Mathematical Models can lead us Astray and What we can do about it, by Erica Thompson
Today I’m reviewing a book that I loved, a book for any actuary, or indeed model builder or model user to read. I felt as if I highlighted half of this book, so I’ll try and give you enough of a flavour of Thompson’s insights so that you feel compelled to read it yourself.
Erica Thompson is a physicist and climate scientist by background. She has taken her background of trying to understand climate atmospheric models (with massively different outputs) for her own research into a broad and rigorous understanding of models – what they can do, what they can’t do, and how to use them most effectively. The title “Escape from Model Land” – is a guide to the philosophy underpinning this book.
Model land is the simplified version of reality that model builders use to illustrate and gain insight into reality. It can never be 100% accurate about all aspects of reality – some parts must be simplified out of existence to make the model even possible. To us a climate example, no climate modellers try to model the colour of the sky, or individual raindrops. But modelling the existence of clouds, and the amount of rain, can be a very useful part of climate modelling, to understand both current weather and future climate. As Thompson says:
The advantage of a mathematical model is the ability to neglect any aspects of the siutation that are not immediately relevant, tractable or important. By removing these aspects, we can reduce the problem to its essence, highlighting only the causal links and developing insight into the behaviour of the subject…The disadvantage of a mathematical model is the necessity of neglecting any aspects of the situation that are not immediately relevant, tractable or important…
…The art of model-making is to draw the boundaries sufficiently wide that we include the important contributing factors, and at the same time sufficiently narrow that the resulting model can be implemented in a useful way.
This of course means that it is really important to understand the purpose of any particular model, as it will almost certainly have problems if used for a purpose that is not the original one. So how do you decide whether any given model can give you reliable, meaningful information about the real world? A particularly important question to think about here is about whether, broadly, the model is an interpolatory model – the model has been built where the observations do not stray much outside the range of the data used to generate the models – or an extrapolatory model – where you are looking to rely on the model to give you information outside the range of available data. Weather models used for forecasting are largely interpolatory, and climate models are largely extrapolatory – we know that the distribution is changing, and we are extrapolating using our understanding of the physical world to answer questions like “what spontaneous changes to social behaviour will occur in the wake of a pandemic?” or “will a species be able to adapt to a changing environmment by changing its diet or behaviour after its normal food becomes unavailable?”
So how do you decide whether a model is fit for purpose? You need to work out what the purpose actually is, first of all. Thompson’s view is that rather than trying to find the single best model of something, we are better off constructing a number of different models, from different angles, and considering their diversity and limitations to inform our thinking. All model building requires value judgements, of which parts of reality are most important for the output, and which variables are likely to be most important to replicate that reality.
The cult of ‘objectivity’ ignores the reality of scientific methods and understanding, and encourages flattening of model information into quantitative metrics rather than informative judgements.
To me this was a key part of the book, that all models require judgement, and blindly applying statistics without understanding of what is being modelled and why is likely to result in a worse model than a combination of expertise in the area being modelled and statistical judgement. Expertise in an area can be biased (and often is) but applying statistics without expertise is likely to result in biases coming from the availability of data or many other sources that are hard to identify. Models are important, and illuminate reality, but it is important to be humble about how good that illumination is, what values are implied, and what gaps remain.
Let’s take the example of the solvency approach to risk management in insurance, which states that insurers must hold capital for the worst event likely to happen in 200 years. An algorithm for deciding what level of capital to set is based around that 1-in-200 year event, which implies a risk attitude and value judgements about the appropriate balance between the risk of not being able to fulfil all contracts versus the profitabiity of insurance businesses. In addition, the model that might be used to determine that 1-in-200- year event size also contains assumptions that amount to value judgements about the acceptable risk of being wrong, and these judgements are made outside Model Land, by the experts who create the model.
I was talking about this very point to a climate actuary this week – how do we know what assumptions are built into our model without explanation of a 1 in 200 year event? If we have a portfolio of buildings that are being insured, what assumptions have we made about the cost of rebuilding. After such an event, two things often happen – builders are scarce and can charge more, and building codes change to help the rebuilt buildings withstand the next event of that type, both of which will increase the cost of rebuilding. How have we allowed for that? What other things might occur that will impact the cost? And of course, what underlying assumptions have been made about the climate in building the model in the first place. Is it today’s climate, or the climate from some other past or future event? Those are the kind of questions that lead you back into the real world, as, while they can be modelled, often asking the questions, rather than hiding assumptions in models, is more helpful. And the actuary or catastrophe modeller building the model, who has made judgements about the data they are using, is more likely to be able to explain these steps back to the real world than relying on someone else who asked a high level question.
Thompson concludes with five principles for responsible modelling, which should be up on the wall in any modelling team:
- Define the purpose – What kinds of questions can this model answer? What kinds of questions can it not answer?
- Don’t say “I don’t know” – the model generally has a point to it, even it is not a perfect representation of reality. if we give up on the prospect of perfect knowledge, what can this model show us about the situation? Does it help us understand tradeoffs between decisions?
- Make value judgements – All models require value judgements. If you can’t find any in your model, look harder, or find someone else to look, who might be affected by the outcome. For example, a model that only looks at economic outcomes of a decision is implicitly not valuing non economic outcomes (such as time that is not paid for by someone).
- Write about the real world – the model builder needs to think about how to translate the outcomes into the real world, not just stay in model land.
- Use many models – this is the principle I found hardest – I instinctively look for the “right” model. But it really does depend on the purpose the model is built for. In particular, using more than one model makes it easier to expose the implicit value judgements that have gone into the building of each one.
And finally,
Although all models are wrong, many are useful…. The future is unknowable, but it is not ungraspable, and the models that we create to manage the uncertainty of the future can play a big role in helping to construct that future.
This was a hard review to write; there is so much insight in this book that it is hard to summarise. Highly recommended.
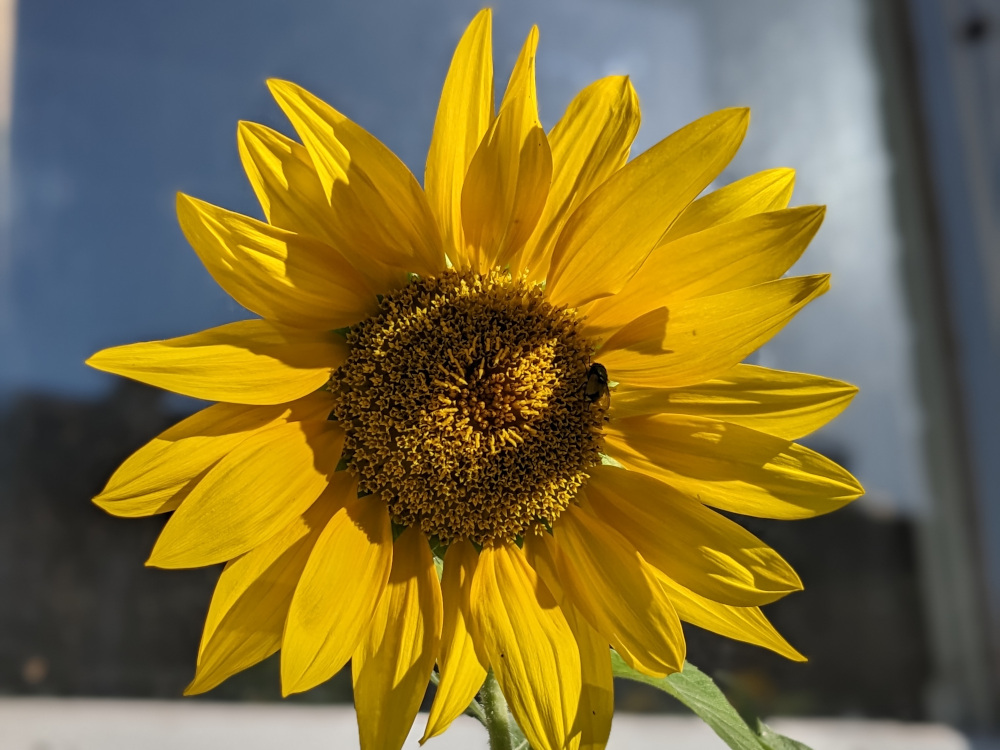
Thanks Jennifer for another worthwhile post and such a compelling recommendation. it sounds relevant in all my fields of interest. It sounds like you felt about this somewhat how I felt about reading The Black Swan by Taleb.
I hope you enjoy it Ian. I also felt the same way about The Black Swan, the other book in that category for me was Thinking Fast and Slow by Daniel Kahneman.